Scaling Generative AI: Laying the Foundation for the Future
- Chandrayee Sengupta
- Nov 15, 2024
- 5 min read
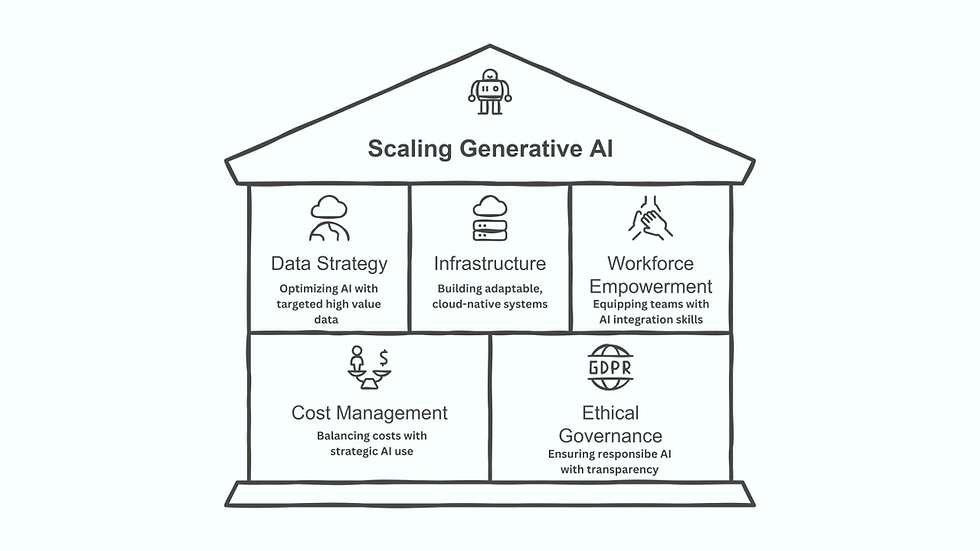
As AI capabilities evolve, organizations are moving beyond pilot programs to full-scale, enterprise-wide generative AI (Gen AI) deployment. Gen AI’s ability to create new content—such as text, images, and videos—brings unique requirements for technical infrastructure, data strategy, workforce empowerment, and responsible governance. This article outlines strategies for scaling Gen AI sustainably, focusing on integrated technical architecture, cost-effective change management, and optimized data practices.
The Power of Data: Fueling Generative AI
Data quality, accessibility, and diversity are essential for scaling Gen AI effectively. Models rely on vast, context-rich data sources to generate accurate and relevant outputs. Rather than striving for data perfection, companies that scale successfully focus on targeting the right data for each use case. By identifying and prioritizing the most valuable data sources and investing in targeted data labeling and authority weighting, organizations can increase Gen AI efficiency and reduce unnecessary costs.
A refined data approach is essential, especially when using techniques like retrieval-augmented generation (RAG), which allows models to pull from specific, high-value sources. Investing in well-curated data—rather than exhaustive data collection—supports Gen AI in delivering high-quality responses without excessive overhead.
Key Metrics to Track Data Strategy:
Data Diversity Levels: Ensures a variety of data sources, improving model robustness and real-world applicability.
Data Freshness and Relevance: Tracks how current and relevant data sources are, enabling the model to adapt to ongoing trends.
Integration Efficiency: Monitors data accessibility and integration speed, minimizing data silos and enhancing model performance.
Building a Unified Data Strategy
A holistic data strategy is the backbone of effective Gen AI scaling. Integrating both structured and unstructured data into a centralized hub enables a seamless flow of information, reducing silos and supporting Gen AI applications with unified, high-quality data access. Organizations should emphasize metrics like data coverage, relevance, and freshness to ensure that models can tap into a reliable, current data pool. By aligning data strategy with specific business goals, companies can unlock data-driven insights that maximize Gen AI’s value.
Infrastructure: Scaling with the Right Architecture
Scaling Gen AI requires an infrastructure built for adaptability, high computational demand, and scalability. Rather than a one-size-fits-all approach, successful Gen AI scaling relies on a modular, cloud-native architecture that allows organizations to support multiple models without redundancies. This architecture incorporates microservices and reusable data pipelines, enabling large-scale data handling and model development.
AI Factories and Modularity: Specialized environments known as AI factories streamline Gen AI development by centralizing data, models, and workflows in a shared space. This modular setup encourages the reuse of assets across different models, creating efficiencies and reducing costs. By leveraging cloud-native, flexible systems, organizations can scale Gen AI capabilities without unnecessary duplication of resources.
Orchestration and End-to-End Automation: To scale Gen AI effectively, infrastructure should support seamless orchestration, allowing multiple models, databases, and applications to work together without performance bottlenecks. End-to-end automation, encompassing everything from data pipeline construction to model monitoring, enables continuous operation while reducing manual intervention. An API gateway or similar orchestration tool can help manage complex workflows, route model requests effectively, and track resource use.
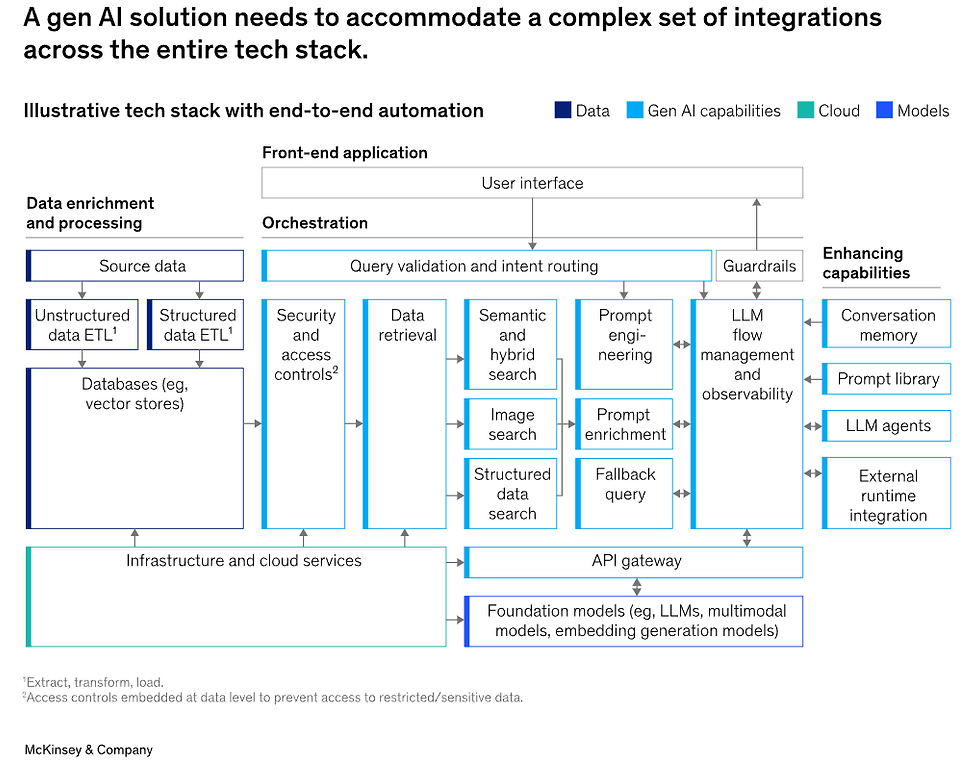
Infrastructure Readiness Metrics:
Compute Resource Utilization: Tracks the demand for processing power, indicating when infrastructure upgrades or optimization are needed.
Cloud Efficiency: Assesses the effectiveness of cloud-based scaling, focusing on latency reduction and cost management.
Scalability Scores: Evaluates how easily the current infrastructure can support rapid scaling across storage, processing, and data needs.
Cost Management: Navigating the Financial Landscape of Gen AI
As organizations scale Gen AI, managing costs becomes crucial. Given the complex infrastructure and high computational requirements of Gen AI, costs can spiral if not managed carefully. Change management, in particular, represents one of the most significant cost factors, often surpassing the initial development costs. For every dollar spent on model development, companies may need to allocate three dollars toward training, adoption, and organizational adjustments to ensure Gen AI applications are fully utilized and aligned with business priorities.
Optimizing Change Management: Successful companies control change management expenses by targeting high-impact use cases, training end-users early, and setting clear KPIs to measure the value Gen AI brings. This focused approach ensures that resources support models that align with critical business needs, maximizing return on investment.
Cost Control in Operations: Running Gen AI models often incurs higher costs than building them, with ongoing expenses like model maintenance, data pipeline updates, and compliance. Cost-reduction strategies—such as using open-source tools where possible, optimizing cloud costs, and reducing unnecessary data collection—help make scaling more sustainable.
Cost-Efficiency Metrics:
Change Management Ratios: Monitors the balance between development and implementation costs.
Run vs. Build Cost Comparison: Tracks ongoing operational expenses relative to initial development, highlighting areas for optimization.
Cloud and Compute Efficiency: Assesses resource utilization for cost-effective scaling.
Empowering the Workforce: Building Value Beyond Technology
Scaling Gen AI effectively requires a workforce that can bridge technology and business needs. Cross-functional teams, built around both technical and business skills, ensure that Gen AI is integrated across departments and not isolated as a technology initiative. Centers of Excellence (COEs) are a proven model for Gen AI scaling, fostering organization-wide AI literacy and enabling departments to leverage Gen AI tools.
Successful organizations invest in continuous workforce training, promoting collaboration across teams and equipping non-technical users to use Gen AI tools confidently. This comprehensive approach to training and knowledge-sharing accelerates adoption and innovation, driving productivity across business units.
Metrics for Workforce Readiness and Collaboration:
Upskilling Completion Rates: Tracks workforce proficiency in Gen AI skills, including MLOps and architecture.
Platform Engagement: Measures how non-technical users interact with AI tools, broadening innovation potential.
Cross-Functional Collaboration Success: Tracks the frequency and effectiveness of cross-departmental Gen AI projects, aligning workforce and technology efforts.
Governance and Ethical Standards: Responsible Scaling
As Gen AI scales, organizations must prioritize ethical governance. Gen AI’s complexity introduces significant privacy and bias risks, requiring ongoing monitoring and transparent practices. Proactive governance helps build stakeholder trust and ensures compliance with evolving AI ethics standards.
Real-Time Monitoring and Transparency: Effective governance includes bias audits, privacy safeguards, and transparency measures embedded into Gen AI models. Automated monitoring tools assess model performance and ethical alignment, ensuring that Gen AI applications operate within acceptable parameters while meeting stakeholder expectations.
Ethical Compliance and Governance Metrics:
Bias Audit Frequency: Conducts regular checks to minimize bias within model outputs.
Privacy and Transparency Scores: Evaluate model explainability and user feedback to foster trust.
Ethical Response Times: Tracks response efficiency to ethical concerns, ensuring alignment with organizational principles.
Conclusion
Scaling Gen AI requires a multi-dimensional approach that integrates technical, operational, and ethical considerations. By focusing on targeted data strategies, flexible infrastructure, cost-efficient management, and a collaborative workforce, organizations can lay a robust foundation for Gen AI at scale. With these pillars in place, companies can unlock the full potential of Gen AI and lead in the dynamic AI landscape, ensuring responsible and sustainable growth.
References
Boston Consulting Group. (2024). The art of scaling GenAI in software. Retrieved from https://www.bcg.com/publications/2024/the-art-of-scaling-genai-in-software
Deloitte AI Institute. (2024). Scaling Generative AI: 13 elements for sustainable growth and value. Deloitte. Retrieved from https://www2.deloitte.com/us/en/pages/consulting/articles/state-of-generative-ai-in-enterprise.html
Google Cloud. (2024). KPIs for Gen AI: Why measuring your new AI is essential to its success. Retrieved from https://cloud.google.com/transform/kpis-for-gen-ai-why-measuring-your-new-ai-is-essential-to-its-success
McKinsey & Company. (2024). Moving past Gen AI’s honeymoon phase: Seven hard truths for CIOs to get from pilot to scale. Retrieved from https://www.mckinsey.com/~/media/mckinsey/business%20functions/mckinsey%20digital/our%20insights/moving%20past%20gen%20ais%20honeymoon%20phase%20seven%20hard%20truths%20for%20cios/moving-past-gen-ais-honeymoon-phase-seven-hard-truths-for-cios-to-get-from-pilot-to-scale.pdf?shouldIndex=false
Qubited. (2024). A data leader’s technical guide to scaling Gen AI. Retrieved from https://qubited.com/a-data-leaders-technical-guide-to-scaling-gen-ai
Smith, J., & Lee, A. (2024). AI hero organizations: Strategies for scaling generative AI across industries. AI Insights Publishing.
Kommentarer